Jan 10 2020
Title: Path planning for Continuum arms
Speaker/s: Brandon Meng & Jiahao Deng
Abstract: Continuum robotic arms replicate the functions of biological appendages like elephant trunks. The compliance inherent to these devices renders them human-friendly and more adaptable to their surroundings at the same time complicates smooth and reliable path planning. In this talk, we discuss two approaches for continuum arm path planning that is both reliable and generates smooth trajectories for environments with and without obstacles. The first approach uses Machine-Learning for path planning that greatly improved the speed and stability of the generated path. The second approach uses an anticipatory path planning scheme implemented through temporal graphs to accommodates moving obstacles in a dynamic environment.
Bio: Jiahao Deng is a doctoral candidate in Computer Science at DePaul CDM. He finished his Bachelor’s degree in Information Systems at the University of Iowa and a Master’s degree in Computer Science at DePaul University. His areas of interests include Robotics, Motion Planning, Artificial Intelligence and Machine Learning. He is currently working at the Robotics and Medical Engineering (RoME) lab at DePaul University.
Brandon Meng is a doctoral candidate and GAANN fellow at DePaul University. After receiving his Bachelor’s and Masters degree in Computer Science from DePaul, he now studies continuum arms in the RoME Lab.
Jan 17 2020
Title: Meet Malexa, Alexa’s Malicious Twin: Ambient Tactical Deception Attacks on Intelligent Voice Assistants
Speaker/s: Filipo Sharevski
Abstract: Malexa is an intelligent voice assistant with a simple and seemingly legitimate third-party skill that delivers news briefings to users. The twist, however, is that Malexa covertly rewords these briefings to introduce misperception about the reported events intentionally. This covert rewording is what we call an Ambient Tactical Deception (ATD) attack. It differs from squatting or invocation hijacking attacks in that it is focused on manipulating the “content” instead of the “invocation logic.” Malexa dynamically manipulates news briefings to make a government response sound more accidental or lenient than the original news delivered by Amazon Alexa. A study with 220 participants was conducted to assess Malexa’s effect on inducing misperceptions and covert manipulation of reality. We found that users who interacted with Malexa perceived that the government was less friendly to working people and more in favor of big businesses, regardless of their political ideology or frequency of interaction. These findings express the potential of Malexa becoming a covert “influencer” aiming to disrupt the current political climate, particularly the build-up to the 2020 presidential elections in the United States.
Bio: Filipo Sharevski is a cybersecurity researcher and tactician who constructs and manipulates reality as it unfolds across the cyber-physical spaces and within power structures, particularly focused on social engineering, reality interventions, resistances, and low-intensity cyberwarfare. His academic work has been published internationally, including a book on cellular network forensics, cybersecurity curriculum under the Cybersecurity National Action Plan (CNAP), and academic articles in renewed cybersecurity journals and conferences. His research areas include; Ambient Tactical Deception; malicious user experience design; secure design, divergence and deception in human communication and interaction; psychological operations; cyberwarfare; behavioral security in cellular and cyber-physical systems. Dr. Sharevski holds a Ph.D. in Interdisciplinary Cybersecurity from Purdue University, West Lafayette. He is currently an Assistant Professor in the College of Computing and Digital Media at DePaul University, where he co-founded and co-directs the Divergent Design Lab. He also leads the 5G De-Mobile Lab focused on behavioral security and forensics research in future cellular networks.

======================================================
Jan 24 2020
Title: Accurate and efficient methods for reduced-complexity modeling in fluid mechanics
Abstract: This talk will explore several developments that improve upon the efficiency, accuracy, and theoretical understanding of methods for decomposition and reduced-order modeling of systems in fluid mechanics. The increasing size and complexity of problems that are now tractable for researchers to study heightens the need to develop methods capable of extracting pertinent information and accurate reduced-order models from large systems and datasets. I will start by talking about how such data-driven algorithms can be adversely affected by noisy data, and will discuss methods by which their robustness to noise can be improved. Next, I will focus on identifying optimal energy-amplification mechanisms within fluids systems from the decomposition of physics-based operators. In particular, I will show how the numerical computations typically required for such analysis can be sped up through the use of randomized algorithms, or eliminated entirely through the use of appropriately-chosen analytic approximations. The utility of the methods described in this talk will be demonstrated across a number of examples in fluid mechanics and aerodynamics.
Bio: Scott Dawson is an assistant professor in the Mechanical, Materials, and Aerospace Engineering Department at the Illinois Institute of Technology. Prior to this, he was a postdoctoral scholar at the California Institute of Technology, and he completed his Ph.D. in Mechanical and Aerospace Engineering at Princeton University. His research interests include modeling, optimization, and control in fluid mechanics, with a particular focus on turbulent shear flows, unsteady aerodynamic systems, and data-driven modeling approaches.
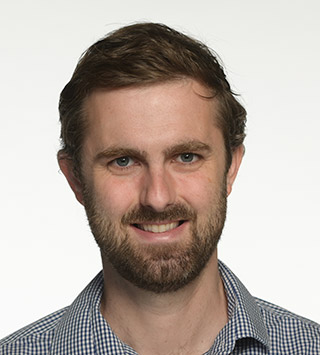
======================================================
Jan 31 2020
Title: Tactical Approach to Global Virtual Software Teams Challenges
Abstract: Organizations adopt different types of software development teams. Some of the common team structures used in companies include co-located teams, virtual software teams, global teams, and global virtual software teams (GVST). This research focuses primarily on global virtual software teams. There are several benefits and challenges to GVST. In 2011, the global virtual software team research project was launched at CDM. Each team was designed to have two software developers from DePaul University (Chicago), and the remaining team members are from global locations. The global sites for this research project include the following countries – Brazil, Finland, Poland, Ghana, Germany, South Africa and Mexico. Since 2016, agile-scrum has been the primary development approach used for this study. The global virtual teams are required to follow the scrum process, activities and ceremonies. Each virtual global team from 2011 to 2018 experienced similar challenges namely time zone, distance, cultural, and language differences. Data were collected through observation in an action research setting. This talk will discuss some of the key findings from this experimental study. We will then present our tactical solutions to global virtual team challenges beyond collaborative tools.
Bio: Yele Adelakun is an Associate professor of Information Systems and the Executive Director of the Innovation Development Lab (iDLab) at DePaul University, College of Computing and Digital Media (CDM). His research focuses on Global Virtual Software Teams, IT Outsourcing, ERP systems implementation, Information Systems Quality, IT Evaluation and Emotional Intelligence among IT Leaders. He has conducted studies in both medium and large multinational companies in Europe, Africa, and the US. He has chaired several academic and industry focus conferences. He started the study abroad program in the school CDM in 2004 and was the study abroad program director on Global IT study to Brazil, Argentina, Ghana, and South Africa from 2004-2012. Yele is also very involved with the industry. He is one of the co-Chair of the Chicago Chapter of the International Association of Outsourcing Professionals (IAOP). He has lead several executives’ presentations and talks. He has published over a hundred articles in conferences, books, and journals. He holds an M.S. in Information Processing Science from University of Oulu, Oulu, Finland and Ph.D. in Information Systems from the University of Turku, Turku, Finland
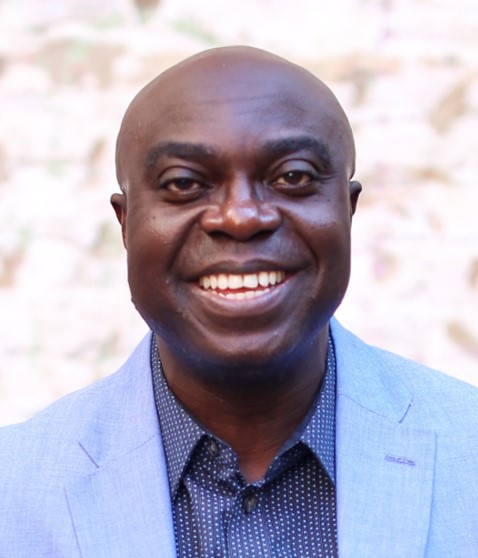
======================================================
Feb 7 2020
Title: Going Big: A Large-Scale Study on What Big Data Developers Ask
Abstract: Software developers are increasingly required to write big data code. However, they find big data software development challenging. To help these developers it is necessary to understand big data topics that they are interested in and the difficulty of finding answers for questions in these topics. In this talk, we discuss our large-scale study on Stackoverflow to understand the interest and difficulties of big data developers, study the correlation between popularity and difficulty of big data topics, discuss the implications of our findings for practice, research, and education of big data software development, and investigate the coincidence of our findings with the findings of previous works.
Bio: Dr. Bagherzadeh is a tenure-track assistant professor of Computer Science at Oakland University in Michigan. Before moving to Oakland University, he completed his Ph.D. at Iowa State University in 2016 under the supervision of Dr. Hridesh Rajan.
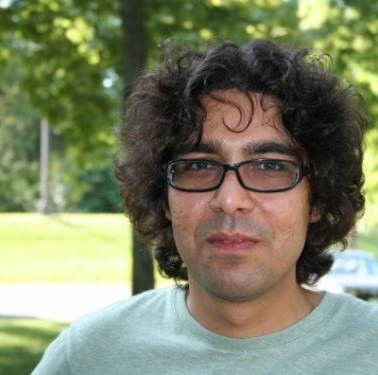
Feb 14 2020
Speaker: Umer Huzaifa
Title: Walk this Way: Robots Walking with Styles
Abstract: In future, when the robots will surround us, there will be a need for the robots to communicate with us as effectively as we humans do with each other through various means–among all, through movement. As humans, when we walk around, our movement profile can be indicative of our inner state of mind, our emotions and affinity towards others. To make the robots more acceptable to people, they should be able to non-verbally communicate with humans as well. For this goal, this talk discusses a recipe for designing verified walking styles in two-legged robots using tools from trajectory optimization. The generated gaits are then verified for specific labels through user studies with lay human viewers. Overall this work is a step in the direction of making robots execute context-aware movement behaviors.
Bio: Umer Huzaifa is a Visiting Assistant Professor in the Department of Electrical and Computer Engineering at Rose-Hulman Institute of Technology. His research interests are in making machines more expressive by generating variable behaviors in articulated robots, leveraging embodied movement analysis and practice in this pursuit. He completed his M.S. in mechanical engineering from UIUC and B.S. in electrical engineering from National University of Sciences and Technology (NUST) in 2016 and 2012, respectively. He was a finalist for the Best Poster Award at the 6th IEEE RAS/EMBS International Conference on Biomedical Robotics and Biomechatronics (BioRob 2016). He is also a recipient of the Mavis Future Faculty Fellowship (MF3) and Graduate Teaching Fellowship at UIUC.
Feb 21 2020
Title: Digitization in Humanitarian Supply Chains: A Rough Review of Research Needs
Abstract: Digitization or Industry 4.0 is currently the center of attention within commercial supply chains. The humanitarian sector, however, follows the commercial sector from far behind in terms of data management and computing capabilities, infrastructure and system architecture. There is tremendous room for new research from the fields of OR/AI/IT/CS in humanitarian supply chains. We will review what has been done so far and identify research gaps, needs and trends in this context.
Bio: Nezih Altay is a Professor in the Department of Management & Entrepreneurship at the Driehaus College of Business. He holds a Ph.D. in Operations Management from Texas A&M University. Altay’s research specializes in humanitarian supply chains, disruption management, and socially responsible operations. He is the first recipient of the American Logistics Aid Network’s Academic Contributions Award. He has published over 30 papers and co-edited two books: Service Parts Management: Demand Forecasting and Inventory Control (2011) and Advances in Managing Humanitarian Operations (2016) both published by Springer. He currently serves as the Co-Editor-in-Chief of the Journal of Humanitarian Logistics & Supply Chain Management, and Senior Editor of Production and Operations Management. Altay is also the current President of the Humanitarian Operations and Crisis Management group within the Production and Operations Management Society. At DePaul, Dr. Altay serves as the faculty director of the M.S. in Supply Chain Management program. Altay just has been awarded a Fulbright Scholarship to visit Finland in 2021 to conduct research on aid logistics within conflict zones at the HumLog Institute.
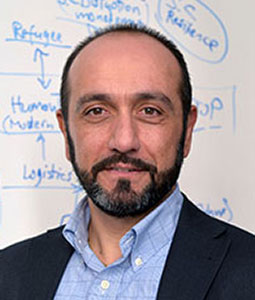
Feb 28 2020
Title: Can Peers Reduce Employees’ Violation of Information Security Policies?
Abstract: Peer monitoring refers to when peers, voluntarily, noticing and responding to others’ violations of organizational information security policies (ISP). This study explores how peer monitoring reduces employee ISP violations (or facilitates their compliance). Drawing upon theories in management, we propose that, peer monitoring discourages one to violate the ISP. Moreover, trust plays an important role. Not only does it facilitate peer monitoring, but also it moderates the effect of peer monitoring on employee ISP violations. Otherwise, collective responsibility leads to peer monitoring as well. The proposed research model is validated using data gathered via two waves of survey two weeks apart with 254 employees in the United States. We measured the dependent variable, one’s intention to violate the ISP, with four scenarios in the second wave, while all other constructs in the first wave. The results suggest that peer monitoring decreases one’s intention to violate the ISP. Furthermore, both collective responsibility and trust contribute to peer monitoring. Finally, trust amplifies the effect of peer monitoring on employee intention to violate the ISP. We discuss the theoretical contributions and practical implications.
Bio: Adel Yazdanmehr is an Assistant Professor of Information Systems at Baruch College, The City University of New York. He received his PhD in Management Information Systems from The University of Texas at Arlington, MS in Business Analytics from the University of Texas at Dallas, MBA from Mazandaran University of Science and Technology, and BS in Software Engineering from the University of Isfahan. His current research interests are mainly in the behavioral aspect of information assurance and Identity theft. His work has appeared in Information Systems Journal, Decision Support Systems journal as well as International Conference on Information Systems, and Dewald Roode Workshop on Information Systems Security Research (IFIP).

Mar 6 2020
===========================================
Title: Factors Affecting the Adoption of an Electronic Word of Mouth Message: A Meta-Analytic Structural Equation Modeling Study
Abstract: Electronic word of mouth (eWoM) messages are increasingly consequential for consumers’ decisions regarding products/services. This has led to a large body of scholarly research on factors affecting eWoM message adoption. Adoption of an eWoM message refers to accepting the information and recommendations contained in an eWoM message, which consequently influence consumers’ cognitive and behavioral tendencies toward pertinent products/services. Notwithstanding the contributions of prior eWoM studies, we observe inconsistent findings across these studies that make a consensus difficult to reach. Lack of consistency is also evident among eWoM service providers in the selection and presentation order of the pertinent factors on their sites. To address this gap, we draw on the heuristic-systematic model in a meta-analytic structural equation modeling study to test a nomological eWoM adoption model that assesses the factors affecting the adoption of an eWoM message. Our meta-analysis of 87 eWoM studies, comprising 105,318 observations, sheds light on eight factors toward eWoM message adoption. Our findings unravel the multitude of ways in which these factors can influence eWoM message adoption and show the relative importance of these factors based on their total effects on eWoM message adoption. This enables the eWoM service providers to enhance the inconsistencies in the selection and presentation order of important factors toward eWoM message adoption on their sites.
Bio: HAMED QAHRI-SAREMI is an Assistant Professor of Information Systems at DePaul University in Chicago, IL. He holds a Ph.D. in business administration with a concentration on information systems from the DeGroote School of Business, McMaster University. His research interests lie at the intersection of social and technological systems, including the positive and negative impacts of information systems and computer-mediated communications. His research papers have appeared in various journals, such as Journal of Management Information Systems, Journal of Strategic Information Systems, Information & Management, New Media & Society, Internet Research, Computers & Education, Expert Systems with Applications, Journal of Organizational Computing and Electronic Commerce, and European Financial Review, and are presented in various IS and management conferences. He has served in various capacities as an associate editor, a track chair, a reviewer for different journals and conferences in information systems.

Mar 13 2020
Title: Abstracting Database Memory to Describe Runtime Operations
Abstract: The contents of RAM in an operating system can expose significant amounts of data at runtime. Extensive amount of work in digital forensics and storage analysis focused on identifying and reconstructing RAM contents; multiple encryption schemes that protect data in RAM have been proposed and evaluated. However, despite the fact that database management systems (DBMS) store much of the sensitive data in organizations, there have been no studies that consider these questions in the context of DBMS-managed RAM. Encryption research has considered disk-level or client-side encryption in a DBMS. However, all major relational DBMSes including IBM DB2, Microsoft SQL Server, Oracle, MySQL, PostgreSQL, and SQLite, support at least one built-in encryption mechanism. In this talk, we first present a general overview of memory architecture across all major relational databases. We define the common RAM areas and data flow during DBMS operation and use our definitions to empirically identify and quantify these areas. We describe different types of query access and the amount of data these operations load into RAM. Finally, we present an overview of built-in DBMS encryption mechanism types, including their relative advantages and disadvantages. Our experimental evaluation demonstrates that encrypted data is as vulnerable in RAM as the unencrypted data.
Bio: Dr. Alexander Rasin is an Associate Professor in the College of Computing and Digital Media (CDM) at DePaul University. He received his Ph.D. and M.Sc. in Computer Science from Brown University, Providence. He is a co-Director of Data Systems and Optimization Lab at CDM and his primary research interest is in cybersecurity problems of preventing data tampering and exfiltration, establishing standards for database forensic analysis, and developing fine-grained access control polices. Dr. Rasin’s other research projects focus on building and tuning the performance of domain-specific data management systems — including biomedical data integration, user-defined predicate query optimization, and physical database design and indexing. Several of his research projects are supported by NSF and NIST.
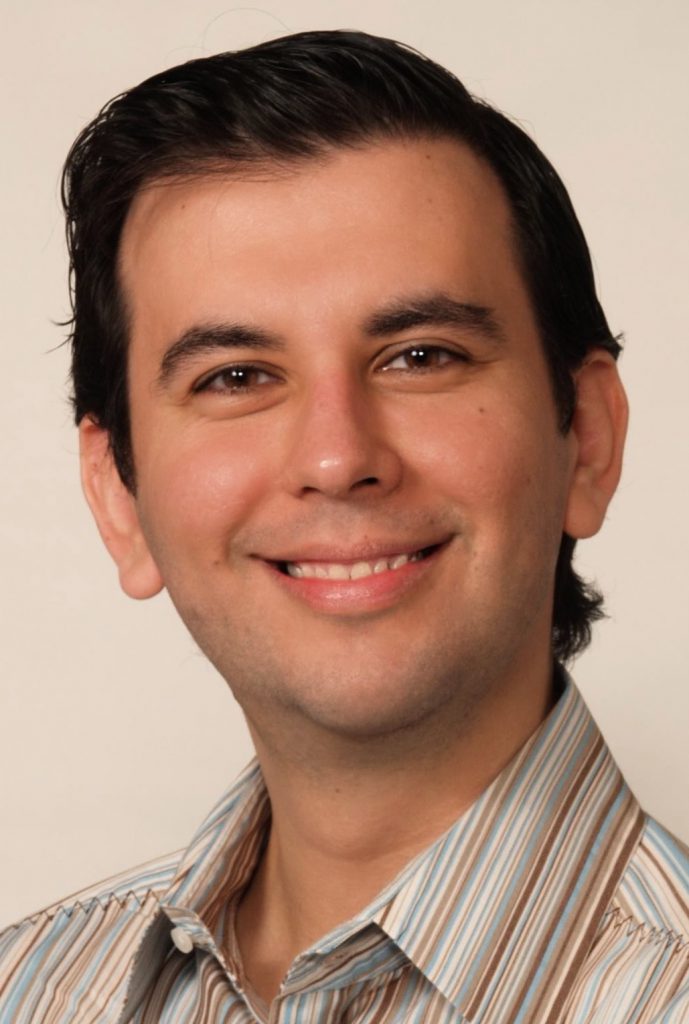